Preliminary Agenda
Preliminary Agenda – Overview
Note: Detailed agenda with information on session topics and presentation schedule will be available in the agenda soon.
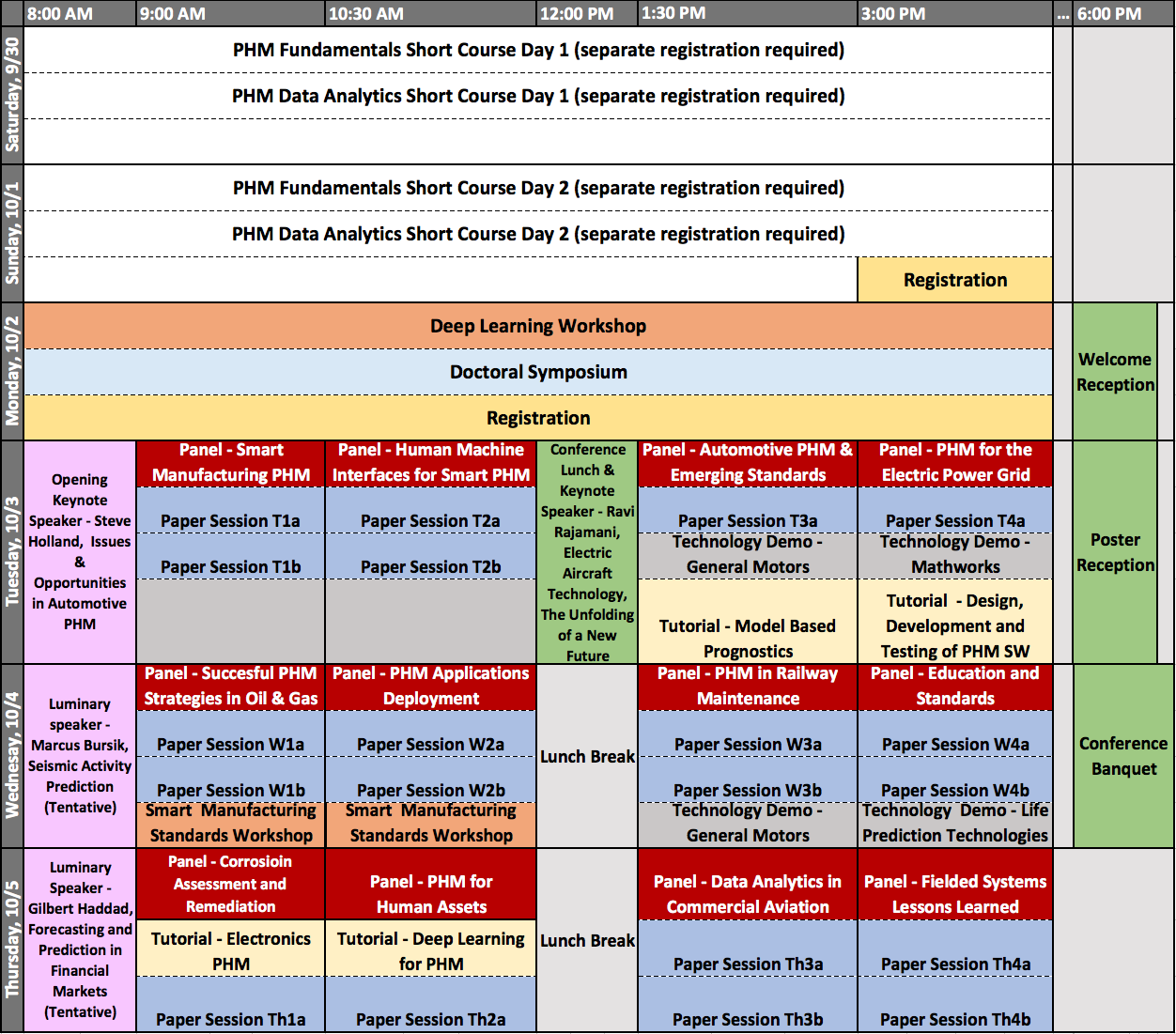
Agenda
<! STYLE INFORMATION, DO NOT ERASE>
<! DAILY SESSION INFO>
Monday, October 2, 2017 — Opening Remarks, Tutorials, Panels, Technical Paper Sessions, Welcome Reception
Tuesday, October 3, 2017 — Luminary, Keynote, Panels, Technical Paper Sessions, Technology Demos, Poster Session
Wednesday, October 4, 2017 — Luminary, Panel, Technical Paper Sessions, Technology Demos, Banquet Dinner
Thursday, October 5, 2017 — Joint PHM/DX Keynote, Technical Paper Sessions, Panel Sessions, Technology Demos, Closing Remarks
Monday, October 2, 2017 | Location | |
---|---|---|
7:00–17:00 | Registration | |
7:00–17:00 | Deep Learning Workshop | St. Petersburg 1 |
7:00–17:00 | Doctoral Symposium | Bayboro |
Tuesday, October 3, 2017 | Location | |
7:00–17:00 | Registration | |
7:30–8:00 | Continental Breakfast | Grand Bay Ballroom and Foyer |
8:00–8:45 | Opening Remarks | Grand Bay Ballroom |
8:00–8:45 | Luminary Presentation: Elaine Spiller, Marquette University | Grand Bay Ballroom |
8:45–10:15 | Session 1A: Batteries I Session Chair: Kai Goebel–NASA Ames Research Center |
St. Petersburg 2 |
Energy Management of Electric Bicycles Given a Traveling Elevation Profile Sebastián Seria1, Vanessa Quintero2, Pablo A. Espinoza3, Aramis Pérez4, Francisco Jaramillo5, Matías Benavides6, Marcos Orchard7– 1,2,3,4,5,6,7 University of Chile, Chile |
||
Use of Teaching-Learning Based Optimization for Filter Parameter Tuning in the Prognostics of a Quadrotor Battery Leonardo Ramos Rodrigues1, João Paulo Pordeus Gomes2, Vandilberto Pereira Pinto3, Roberto Kawakami Harrop Galvão4– 1 Institute of Aeronautics and Space, Brazil 2,3 Federal University of Ceará, Brazil 4 Instituto Tecnológico de Aeronáutica, Brazil |
||
GPU Accelerated Prognostics George E. Gorospe1, Matthew J. Daigle2, Shankar Sankararaman3, Chetan S. Kulkarni4, Eley Ng5– 1,3,4 SGT Inc., NASA Ames Research Center 2 NIO USA, Inc. 5 Universities Space Research Association |
||
Lithium-ion Battery Remaining Useful Life Prediction with Long Short-term Memory Recurrent Neural Network Yuefeng Liu1, Guangquan Zhao2, Xiyuan Peng3, Cong Hu4– 1,2,3 Harbin Institute of Technology, China 1 Inner Mongolia University of Science & Technology, Inner Mongolia 4 Guilin University of Electronic Technology, China |
||
8:45–10:15 | Session 1B: Industrial & Manufacturing Applications Session Chair: Francesco Cannarile–Politecnico di Milano |
St. Petersburg 3 |
Fleet Knowledge for Prognostics and Health Management – Identifying Fleet Dimensions and Characteristics for the Categorization of Fleets Carolin Wagner1, Bernd Hellingrath2– 1,2 Westfälische Wilhelms-Universität Münster, Germany |
||
Dynamic Modeling of Maintenance Prices in the Aerospace Industry George S. Ekladious1, Xiaojun Zhao2, Hala Mostafa3, Ramona Georgescu4– 1,2,3,4 United Technologies Research Center |
||
Case Studies in using Consumer Analytics with PHM Strategy Sameer Vittal1, Mark Sporer2– 1 GE Power 2 GE Renewables |
||
A New Application for Failure Prognostics – Reduction of Automotive Electronics Reliability Test Duration Andre Kleyner1, Arvind Vasan2, Michael Pecht3– 1 Delphi Electronics & Safety 2 Empower Micro Systems Inc. 3 CALCE Research Center, University of Maryland |
||
8:45–10:15 | Panel Session 1: Smart Manufacturing PHM | St. Petersburg 1 |
10:15–10:30 | Break | St. Petersburg Foyer |
10:30–12:00 | Session 2A: Batteries II Session Chair: Chetan Kulkarni–SGT Inc., NASA Ames Research Center |
St. Petersburg 2 |
Flight Tests of a Remaining Flying Time Prediction System for Small Electric Aircraft in the Presence of Faults Edward F. Hogge1, Chetan S. Kulkarni2, Sixto L. Vazquez3, Kyle M. Smalling4, Thomas H. Strom5, Boyd L. Hill6, Cuong C. Quach7– 1,4,5 Northrop Grumman Technology Services 2 SGT, Inc., NASA Ames Research Center 1,3,4,5,6,7 NASA Langley Research Center 6 Analytical Mechanics Associates, Inc. |
||
A Simulation Engine for Predicting State-of-Charge and State-of-Health in Lithium-Ion Battery Packs of Electric Vehicles Pablo A. Espinoza1, Aramis Pérez2, Marcos E. Orchard3, Hugo F. Navarrete4, Daniel A. Pola5– 1,2,3,4,5 University of Chile, Chile |
||
An Improved Model for Remaining Useful Life Prediction on Capacity Degradation and Regeneration of Lithium-ion Battery Li-Ming Deng1, Yu-Cheng Hsu2, Han-Xiong Li3– 1,2,3 City University of Hong Kong, China |
||
10:30–12:00 | Session 2B: Deep Learning Session Chair: Neil Eklund–Analatom |
St. Petersburg 3 |
Deep Feature Learning Network for Fault Detection and Isolation Gabriel Michau1, Thomas Palmé2, Olga Fink3– 1,3 Zurich University of Applied Sciences, Switzerland 2 General Electric (GE), Switzerland |
||
Unsupervised Deep Learning for Gear Health Monitoring Tyler Cody1, Stephen Adams2, Peter A. Beling3– 1,2,3 University of Virginia |
||
Bearing Health Condition Prediction Using Deep Belief Network Guangquan Zhao1, Xiaoyong Liu2, Bin Zhang3, Guohui Zhang4, Guangxing Niu5, Cong Hu6– 1,2,4 Harbin Institute of Technology, China 3,5 University of South Carolina 6 Guilin University of Electronic Technology, China |
||
10:30–12:00 | Panel Session 2: Human Machine Interfaces for Smart PHM | St. Petersburg 1 |
12:00–13:15 | Conference Lunch and Keynote Speech – Ravi Rajmani, drR2 Consulting | Grand Bay Ballroom |
1:15–3:00 | Session 3A: Wind Turbines Session Chair: Jason Deutsch–University of Illinois, Chicago |
St. Petersburg 2 |
Adaptive Training of Vibration-based Anomaly Detector for Wind Turbine Condition Monitoring Takanori Hasegawa1, Jun Ogata2, Masahiro Murakawa3, Tetsunori Kobayashi4, Tetsuji Ogawa5– 1,4,5 Waseda University, Japan 1,2,3,5 National Institute of Advanced Industrial Science and Technology, Japan |
||
Wind Turbine Intelligent Gear Fault Identification Sofia Koukoura1, James Carroll2, Alasdair McDonald3– 1,2,3 University of Strathclyde, UK |
||
Small-Scale Wind Turbine Recurrence and Cost Modeling as a Function of Operational Covariates from Supervisory Control and Data Acquisition Systems Michael S. Czahor1, William Q. Meeker2– 1,2 Iowa State University |
||
13:15–15:00 | Tutorial Session 1: Model-Based Prognostics – An Introduction, Indranil Roychoudhury, SGT Inc., NASA Ames Research Center | St. Petersburg 3 |
13:15–15:00 | Panel Session 3: Automotive PHM and Emerging Standards, Steven W. Holland, General Motors | St. Petersburg 1 |
13:15–15:00 | Technology Demonstration: Automotive Application of PHM Concepts via Cadillac SRX Rig [General Motors] | Williams and Demens |
15:00–15:30 | Break | St. Petersburg Foyer |
3:30–5:00 | Session 4A: Diagnostics Session Chair: Michael Sharp–NIST |
St. Petersburg 2 |
Diagnostics of machine tool linear axes via separation of geometric error sources Gregory W. Vogl1, Michael E. Sharp2– 1,2 National Institute of Standards and Technology |
||
Towards Diagnosing Cascading Outages in Cyber Physical Energy Systems using Temporal Causal Models Ajay Chhokra1, Nagabhushan Mahadevan2, Abhishek Dubey3, Daniel Balasubramanian4, Gabor Karsai5– 1,2,3,4,5 Vanderbilt University |
||
Internet of Turbines: An Outlook on Smart Diagnostics Gulnar Mehdi1, Mikhail Roshchin2, Thomas Runkler3– 1,2,3 Siemens AG, Germany 1,3 Technical University Munich, Germany |
||
Diagnosis and Prognosis of Fuel Injectors based on Control Adaptation Azeem Sarwar1, Chaitanya Sankavaram2, Xiangxing Lu3– 1,2,3 General Motors Company |
||
15:30–17:00 | Tutorial Session 2: Design, Development, and Testing of PHM Software, Chris Teubert, NASA Ames Research Center | St. Petersburg 3 |
15:30–17:00 | Panel Session 4: PHM for the Electric Power Grid, Avi Gopstein, NIST | St. Petersburg 1 |
15:30–17:00 | Technology Demonstration: Machine Health Monitoring via Internet of Things Platform [Mathworks] | Williams and Demens |
18:00–19:30 | Cocktail Reception with Posters | Grand Bay Ballroom |
Wednesday, October 4, 2017 | Location | |
7:00–17:00 | Registration | |
7:30–8:00 | Continental Breakfast | Grand Bay Ballroom and Foyer |
8:00–8:45 | Opening Remarks | Grand Bay Ballroom |
8:00–8:45 | Keynote Speaker, Steve Holland, Issues and Opportunities in Automotive PHM | Grand Bay Ballroom |
8:45–10:15 | Session 5A: Aviation I Session Chair: Karl Reichard–Pennsylvania State University |
St. Petersburg 2 |
Fast Optimization for aircraft Descent and Approach Trajectory Dmitry G. Luchinskiy1, Stefan Schuet2, J. Brenton3, Dogan Timucin4, David Smith5, John Kaneshige6– 1 SGT, Inc. 2,3,4,5,6 NASA Ames Research Center |
||
Health-Informed Uncertainty Quantifications via Bayesian Filters with Markov Chain Monte Carlo Simulations for Fatigue Critical Rotorcraft Components Michael Shiao1, Anindya Ghoshal2– 1,2 Army Research Laboratory |
||
Reducing the Impact of Test Bench Component on the Thrust Margin Measurement Mohammed Meqqadmi1, Pierre-Etienne Mosser2, Thierry Brichler3, Jérôme Lacaille4– 1,2,3,4 Safran Aircraft Engines, France |
||
8:45–10:15 | Session 5B: Data Driven Methods I Session Chair: Jamie Coble–University of Tennessee, Knoxville |
St. Petersburg 3 |
A Method for Measuring the Robustness of Diagnostic Models for Predicting the Break Size during LOCA Xiange Tian1, Victor Becerra2, Nils Bausch3, Gopika Vinod4, T.V. Santhosh5– 1,2,3 University of Portsmouth, UK 4,5 Bhabha Atomic Research Centre, India |
||
Condition Monitoring of a Reciprocating Compressor Using Wavelet Transformation and Support Vector Machines Shawn Falzone1, Jason R. Kolodziej2– 1,2 Rochester Institute of Technology |
||
Data Driven Modeling and Estimation of Accumulated Damage in Mining Vehicles using On-board Sensors Erik Jakobsson1, Erik Frisk2, Robert Pettersson3, Mattias Krysander4– 1,3 Atlas Copco Rock Drills AB, Sweden 1,2,4 Linköping University, Sweden |
||
Fault Detection By Segment Evaluation Based On Inferential Statistics For Asset Monitoring Vepa Atamuradov1, Kamal Medjaher2, Benjamin Lamoureux3, Pierre Dersin4, Noureddine Zerhouni5– 1,2 INP-ENIT, France 3,4 ALSTOM Transport, France 5 FEMTO-ST Institute, France |
||
8:45–10:15 | Smart Manufacturing Standards Worshop | Bayboro |
8:45–10:15 | Invited Session: Data Challenge Winners | St. Petersburg 1 |
10:15–10:30 | Break | St. Petersburg Foyer |
10:30–12:00 | Session 6A: Aviation II Session Chair: Yuan Di–University of Cincinnati |
St. Petersburg 2 |
A Case for the Use of Data-driven Methods in Gas Turbine Prognostics Marcia Baptista1, Cairo L. Nascimento2, Helmut Prendinger3, Elsa Henriques4– 1,4 Universidade de Lisboa, Portugal 2 Instituto Tecnologico de Aeronautica, Brazil 3 National Institute of Informatics, Japan |
||
Effect of Ambient Temperature on Performance of Gas Turbine Engine Yuan Liu1, Avisekh Banerjee2, Amar Kumar3, Alka Srivastava4, Nita Goel5– 1,2 Life Prediction Technology Inc., Canada 3,4,5 Tecsis Corporation, Canada |
||
Prospective Architectures for Onboard vs Cloud-based Decision Making for Unmanned Aerial Systems Shankar Sankararaman1, Christopher Teubert2– 1 SGT, Inc. 1,2 NASA Ames Research Center |
||
10:30–12:00 | Session 6B: Data Driven Methods II Session Chair: Mohamed Ismail–Institute of Flight Systems, German Aerospace Center (DLR) |
St. Petersburg 3 |
A Compressed Sensing Feature Extraction Approach for Diagnostics and Prognostics in Electromagnetic Solenoids Christian Knoebel1, Hanna Wenzl2, Johannes Reuter3, Clemens Guehmann4– 1,2,3 University of Applied Sciences Konstanz, Germany 4 Technische Universität Berlin, Germany |
||
Fault Detection and Prognosis of Time Series Data with Random Projection Filter Bank Sepideh Pourazarm1, Amir-massoud Farahmand2, Daniel Nikovski3– 1 Mitsubishi Electric Research Laboratories |
||
A Comparison of Acoustic Emission and Vibration Measurements for Condition Monitoring of an Offshore Drilling Machine Martin Hemmer1, Tor I. Waag2– 1 University of Agder, Norway 2 Teknova AS, Norway |
||
10:30–12:00 | Smart Manufacturing Standards Worshop | Bayboro |
10:30–12:00 | Panel Session 5: PHM Applications Deployment | St. Petersburg 1 |
12:00–13:15 | Lunch on Your Own | |
1:15–3:00 | Session 7A: Systems I Session Chair: George Gorospe–SGT Inc., NASA Ames Research Center |
St. Petersburg 2 |
Inferential Framework for Autonomous Cryogenic Loading Operations Dmitry G. Luchinskiy1, Michael Khasin2, Dogan Timucin3, Jarred Sass4, Jose Perotti5, Barbara Brown6– 1,2 SGT, Inc. 3 NASA Ames Research Center 4,5,6 Kennedy Space Center |
||
Integration of Prognostics at a System Level: a Petri Net Approach Manuel Chiachío1, Juan Chiachío2, Shankar Sankararaman3, John Andrews4– 1,2,4 University of Nottingham, UK 3 NASA Ames Research Center |
||
Why Autonomous Assets are Good for Reliability – the Impact of ‘Operator-related Component’ Failures on Heavy Mobile Equipment Reliability Melinda R. Hodkiewicz1, Zac Batsioudis2, Tyler Radomiljac3, Mark T.W. Ho4– 1,2,3,4 University of Western Australia, Australia |
||
PFsuper: Simulation-Based Prognostics to Monitor and Predict Sparse Time Series Javier Echauz1, Andrew Gardner2, Ryan R. Curtin3, Nikolaos Vasiloglou4, George Vachtsevanos5– 1,2,3,4 Symantec Corporation 5 Georgia Institute of Technology |
||
1:15–3:00 | Session 7B: Structural Health Management Session Chair: Juan Chiachio–University of Nottingham |
St. Petersburg 3 |
Low Computation Acoustic Emissions Structural Health Monitoring Through Analog Signal Pre-Processing Rune Schlanbusch1, Eric Bechhoefer2, Thomas J. J. Meyer3– 1,3 Teknova, Norway 2 GPMS Inc. |
||
Low-complexity Behavioral Model for Predictive Maintenance of Railway Turnouts Pegah Barkhordari1, Roberto Galeazzi2, Alejandro de Miguel Tejada3, Ilmar F. Santos4– 1,2,3,4 Technical Univerisity of Denmark, Denmark |
||
Impact Damage Prediction for Wave Energy Converters Ryan Meekins1, Stephen Adams2, Kevin Farinholt3, Nathan Hipwell4, Michael Desrosiers5, Peter Beling6– 1,2,6 University of Virginia 3,4,5 Luna Innovations, Inc. |
||
Preliminary Results on Condition Monitoring of Fiber Ropes using Automatic Width and Discrete Length Measurements Shaun Falconer1, Andreas Gromsrud2, Espen Oland3, Geir Grasmo4– 1,2,4 University of Agder, Norway 3 Teknova AS, Norway |
||
13:15–15:00 | Panel Session 6: PHM in Railway Maintenance, Parham Shahidi, PARC | St. Petersburg 1 |
13:15–15:00 | Technology Demonstration: Automotive Application of PHM Concepts via Cadillac SRX Rig [General Motors] | Williams and Demens |
15:00–15:30 | Break | St. Petersburg Foyer |
3:30–5:00 | Session 8A: Systems II Session Chair: Roghieh Abdollahi–Clemson University |
St. Petersburg 2 |
Gear Fault Diagnostics Using Extended Phase Space Topology T. Haj Mohamad1, C. Nataraj2– 1,2 Villanova Center for Analytics of Dynamical Systems |
||
Actuator Fault-Detection for Autonomous Underwater Vehicles Using Unsupervised Learning Matt Kemp1, Ben Raanan2– 1,2 Monterey Bay Aquarium Research Institute |
||
Unobtrusive Software and System Health Management with R2U2 on a parallel MIMD Coprocessor Johann Schumann1, Patrick Moosbrugger2– 1 SGT, Inc., NASA Ames Research Center 2 Vienna University of Technology, Austria |
||
3:30–5:00 | Session 8B: Anomaly Detection Session Chair: Dmitry Luchinsky–SGT Inc., NASA Ames Research Center |
St. Petersburg 3 |
Anomaly Detection Using Dynamical Linear Models and Sequential Testing on a Marine Engine System Erik Vanem1, Geir Olve Storvik2– 1 DNV GL, Norway 1,2 University of Oslo, Norway |
||
Leak Detection in Compressed Air Systems using Unsupervised Anomaly Detection Techniques Antoine Desmet1, Matthew Delore2– 1 Komatsu Mining Corporation, Australia 2 University of Newcastle, Australia |
||
Early Warnings for failing Train Axle Bearings based on Temperature M.F.E. Peters1– 1 Netherlands Railways (NS), Nederland |
||
15:30–17:00 | Panel Session 7: PHM Education and Standards, Jeff Bird (TECnos) and Ravi Rajmani (drR2 Consulting) | St. Petersburg 1 |
15:30–17:00 | Technology Demonstration: Model-based Predictive Maintenance Solutions for Specific Turbine Engines [Life Prediction Technologies] | Williams and Demens |
18:00–22:00 | PHM Conference Banquet | Museum of Fine Arts |
Thursday, October 5, 2017 | Location | |
7:00–17:00 | Registration | |
7:30–8:00 | Continental Breakfast | Grand Bay Ballroom and Foyer |
8:00–8:45 | Opening Remarks | Grand Bay Ballroom |
8:00–8:45 | Luminary Presentation Gilbert Haddad, Sparkcognition, Digital Transformation Across Industries: Is PHM only for Industrial Assets? | Grand Bay Ballroom |
8:45–10:15 | Session 9A: Standards & Methodologies Session Chair: Carolin Wagner–University of Münster |
St. Petersburg 2 |
Identification of Industrial Robot Arm Work Cell Use Cases and a Test Bed to Promote Monitoring, Diagnostic, and Prognostic Technologies Brian A. Weiss1, Alexander Klinger2– 1 National Institute of Standards and Technology |
||
Trends in Research Techniques of Prognostics for Gas Turbines and Diesel Engines Joseph T. Bernardo1, Karl M. Reichard2– 1,2 The Pennsylvania State University Applied Research Laboratory |
||
The Role of Transactional Data in Prognostics and Health Management Work Processes Sarah Lukens1, Manjish Naik2, Xiaohui Hu3, Donald S. Doan4, Shaddy Abado5– 1,2,3,4,5 GE Digital |
||
A Generic Software Architecture for Prognostics Christopher Teubert1, Matthew Daigle2, Shankar Sankararaman3, Kai Goebel4, Jason Watkins5– 1,2,3,4 NASA Ames Research Center 3 SGT, Inc. 5 University of California, |
||
8:45–10:15 | Tutorial Session 3: Electronics PHM, Patrick Kalgren, Singularity – Intelligence Amplified | St. Petersburg 3 |
8:45–10:15 | Panel Session 8: Corrosion Assessment and Remediation, Edward Manns (NACE) | St. Petersburg 1 |
10:15–10:30 | Break | St. Petersburg Foyer |
10:30–12:00 | Session 10A: Bearings PHM Session Chair: Manuel Chiachio–University of Nottingham |
St. Petersburg 2 |
Challenges And Opportunities in Applying Vibration Based Condition Monitoring in Railways Diego A. Tobon-Mejia1, Pierre Dersin2, Gerard Tripot3– 1,2,3 ALSTOM, France |
||
Steps Toward Prognostics of Faults in Bearings Eyal Madar1, Gideon Kogan2, Dmitri Gazizulin3, Renata Klein4, Jacob Bortman5– 1,2,3,5 Ben-Gurion University of the Negev, Israel 4 R.K. Diagnostics, Israel |
||
Feature Extraction for Bearing Prognostics using Correlation Coefficient Weight Seokgoo Kim1, Chaeyoung Lim2, Joo-Ho Choi3– 1,2,3 Korea Aerospace University, Korea |
||
Condition Based Maintenance of Low Speed Rolling Element Bearings using Hidden Markov Model Guru Prakash1, Sriram Narasimhan2, Mahesh Pandey3– 1,2,3 University of Waterloo, Canada |
||
10:30–12:00 | Tutorial Session 4: Deep Learning for PHM, Emilien Dupont, Schlumberger | St. Petersburg 3 |
10:30–12:00 | Panel Session 9: PHM for Human Assets, Wolfgang Fink, University of Arizona | St. Petersburg 1 |
12:00–13:15 | Lunch on Your Own | |
1:15–3:00 | Session 11A: Railway PHM Session Chair: Jeff Bird–TECnos Consulting Services |
St. Petersburg 2 |
Combination of Data-driven Feature Selection Methods with Domain Knowledge for Diagnosis of Railway Vehicles Bernhard Girstmair1, Andreas Haigermoser2, Justinian Rosca3– 1,2 SIEMENS AG, Austria 3 SIEMENS Corporate Technology |
||
A Reliability-based Prognostics Framework for Railway Track Management Juan Chiachío1, Manuel Chiachío2, Darren Prescott3, John Andrews4– 1,2,3,4 University of Nottingham, UK |
||
Some Influencing Factors for Passenger Train Punctuality in Sweden Carl-William Palmqvist1, Nils O. E. Olsson2, Lena Winslott Hiselius3– 1,2,3 Lund University, Sweden 2 Norwegian University of Science and Technology, Norway |
||
Anomaly Detection and Severity Prediction of Air Leakage in Train Braking Pipes Wan-Jui Lee1– 1 Dutch Railways, Delft University of Technology, the Netherlands |
||
1:15–3:00 | Session 11B: Prognostics I Session Chair: Abbas Chokor–Seagate Technologies |
St. Petersburg 3 |
HPPN-based Prognosis for Hybrid Systems Pauline Ribot1, Elodie Chanthery2, Quentin Gaudel3– 1,2 LAAS-CNRS, Université de Toulouse, France 3 Easymile, France |
||
A Condition Based Maintenance Implementation for an Automated People Mover Gearbox Ali Ashasi-Sorkhabi1, Stanley Fong2, Guru Prakash3, Sriram Narasimhan4– 1,2,3,4 University of Waterloo, Canada |
||
13:15–15:00 | Panel Session 10: Data Analytics in Commercial Aviation, Rhonda Walthall, UTC Aerospace Systems | St. Petersburg 1 |
15:00–15:30 | Break | St. Petersburg Foyer |
3:30–5:00 | Session 12A: Electronics PHM Session Chair: Ian Jennions–Cranfield University |
St. Petersburg 2 |
Application of a Relative Humidity Sensor for Monitoring Water Vapor Concentration inside Enclosures Brian Hatchell1, Eric Gonzales2, Anton Sinkov3, Lorenzo Luzi4, Azem Cakerri5– 1,2,3,4 Pacific Northwest National Laboratory 5 U.S. Army ARDEC |
||
Prognosis of Connector Disconnection Using a Canary-Based Approach Xinyu Du1, Atul Nagose2, Aaron Bloom3, Timothy Julson4– 1,2,3,4 General Motors |
||
Impact of Modulation Frequencies on the Lifetime of Power Semiconductor Modules for EV Applications Quentin Gestes1, Nicolas Degrenne2– 1 Ecole Normale Superieure de Rennes, France 2 Mitsubishi Electric R&D Centre Europe, France |
||
An Observer-based On-line Electrolytic Capacitor Health Monitoring System Laurent Foube1– 1 Mitsubishi Electric R&D Centre Europe, France |
||
3:30–5:00 | Session 12B: Prognostics II Session Chair: Stephen Adams–University of Virginia |
St. Petersburg 3 |
Spatio-temporal Probabilistic Modeling Based on Gaussian Mixture Models and Neural Gas Theory for Prediction of Criminal Activity Francisco Jaramillo1, Vanessa Quintero2, Aramis Pérez3, Marcos Orchard4– 1,2,3,4 Universidad de Chile, Chile |
||
A Comparison of Feature Selection and Feature Extraction Techniques for Condition Monitoring of a Hydraulic Actuator Stephen Adams1, Ryan Meekins2, Peter A. Beling3, Kevin Farinholt4, Nathan Brown5, Sherwood Polter6, Qing Dong7– 1,2,3 University of Virginia 4,5 Luna Innovations Inc. 6,7 Naval Surface Warfare Center |
||
Improvement of a Hydrogenerator Prognostic Model by using Partial Discharge Measurement Analysis Mélanie Lévesque1, Normand Amyot2, Claude Hudon3, Mario Bélec4, Olivier Blancke5– 1,2,3,4 Institut de Recherche d’Hydro-Québec, Canada 5 École de Technologie Supérieure, Canada |
||
A Bi-Level Weibull Model with Applications to Two Ordered Events Shuguang Song1, Hanlin Liu2, Mimi Zhang3, Min Xie4– 1 The Boeing Company 2,4 City University of Hong Kong, Hong Kong SAR 3 University of Strathclyde, UK |
||
15:30–17:00 | Panel Session 11: Fielded Systems Lessions Learned, Andy Hess, The Hess PHM Group | St. Petersburg 1 |
17:00–17:30 | Closing Remarks | St. Petersburg 1 |